Dr. Jonathan Perlin Discusses How Patients Benefit From the Use of Big Data
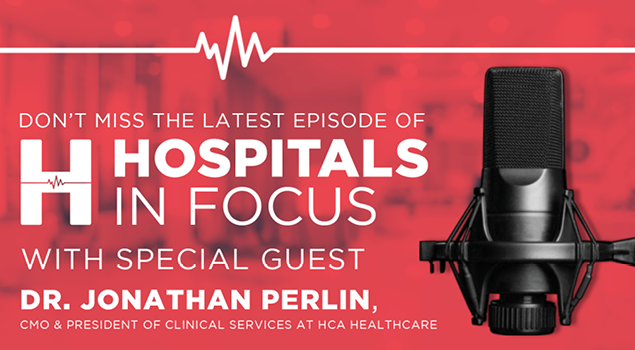
Medicine, technology and data science have merged in the 21st century. Using big data, hospitals and machines have come together to achieve better health outcomes for patients. In this episode, Chip talks with Dr. Jonathan Perlin, CMO and President of Clinical Services at HCA. They discuss how HCA’s size and scale is helping to collect, analyze and act on the wealth of information being collected in the hospital.
Chip Kahn (00:11):
Today, we are in Nashville at the headquarters of HCA to learn more about how the company uses its scale and so-called big data to help it revolutionary patient care and ultimately get better outcomes. And with the advances are that could affect the healthcare for Americans across the country, HCA is one of the nation’s leading providers of healthcare services and is made up of locally managed facilities that include 178 hospitals, and 119 freestanding surgery centers, located in 20 US states, and also has hospitals in the United Kingdom. Our guest today is doctor Jon Perlin, the president of clinical services and chief medical officer for HCA. He joined the company in 2006. He also recently was appointed to the Medicare Payment Advisory Commission which advises the Congress on Medicare matters. Before joining HCA, Dr. Perlin served as the Under Secretary for health at the Veterans Administration. It is just one of the many vital roles he has held in our industry and the health care system generally.
But we are here today to focus our talk about things he is working on currently at HCA, driving value for healthcare services for patients through big data science and advanced analytics, and ultimately using what they have learned to improve outcomes for patients. Dr. Perlin has three main headline grabbing areas here that we’ll begin our discussion with from work at HCA. HCA has used its size and scale to make big discoveries and ultimately big changes in patient care. Some have affected better outcomes for newborns, others the spread of MRSA infections, and finally the reduction of Sepsis, one of the major life threatening infections that patients can suffer from.
So Dr. Perlin, give us a sense for why HCA and why these kinds of advances we’re going to discuss could be done in an organization, a system like yours.
Dr. Jon Perlin (02:33):
Well first Chip, let me just say what a privilege it is to be here with you and thanks for the opportunity to help us share why learning is so important to our organization. You know, the opportunity of scale isn’t just to have more size, it’s to really accelerate learning and improvement. A few years back when meaningful use was a requirement, that is a requirement to computerize the hospital system entirely, we said, “What is our real opportunity here? Is it just to replace with some newer electronic health records or is it to lay in the footprint of a learning health system?” And by learning health system, we really made a system in which the inevitable data byproduct of every patient encounter is collected in such a way that it can inform and improve every subsequent patient encounter, even for that same patient by feeding real time decision support.
It also means that those same data can help to inform the system by channeling back in as information to improve the safety, the quality, the efficiency, and even the compassion of care. We view this as both a privilege and a responsibility and we’re pleased to have been able to rally the data byproduct of more than 30 million patient encounters every year that we’re privileged to provide into helping better inform improvements in outcome, and childbirth, the prevention of life threatening hospital acquired infections, and the control, as you mentioned of Sepsis, really one of the most challenging diagnoses that can afflict patients in hospitals. It’s really an overwhelming infection. We’re using artificial intelligence and data science to help to identify Sepsis early. I look forward to discussing each of these with you.
Chip Kahn (04:19):
Jon, let’s first focus on newborns. Can you tell us why 39 weeks is such a significant point of departure and what would the problems that we’ve been experiencing in the birthing process for those that have been born previously prior to that point?
Dr. Jon Perlin (04:41):
Well, the issue of childbirth at 39 weeks is a really interesting one. Against the backdrop of all of evolution, in the past 35 to 40 years, humans have shortened, in the United States, the length of time that mom carries baby to full term. Full term we always thought of as 39 weeks, but with parental preference, a birthday, a special day, and physician preference, we had over that period of time reduced by 10 days the length of time women can carry babies to term. Now, babies have to be really robust. You know, against all of evolution, the saber tooth tiger or whatever the threat was, they had to survive, and so no hospital, let alone an individual obstetrician, noticed much of a difference between babies that were born early, that is electively early, not when mom or baby were in distress, at 37, 38, or 39 weeks.
But there are some reports that maybe there is a difference. And so, The March of Dimes came to HCA and said, “Hey, you all deliver a lot of babies.” And the truth is we deliver an awful lot of babies, over 200,000 babies a year, about two thirds as many babies who are born in Australia. They said, “I bet you could use your platform to answer this question of whether there’s a difference between 37, 38, and 39 weeks of pregnancy.”
We thought about how we might do this, and so we got 27 hospitals together and over the course of 90 days looked at the results of nearly 18,000 deliveries, 17,794 to be precise, and going to the newborn intensive care unit as a proxy for something bad, something bad being something like inability to feed or respiratory distress. We looked at the differences in rates of going to the newborn intensive care unit.
Turns out the signal wasn’t subtle. The risk for something bad and going to the newborn intensive care unit for elective delivery was four times greater at 37 weeks than 39 weeks, and over twice as great at 38 versus 39 weeks, suggesting that unless mom or baby were in distress, mother nature had it right. The length of pregnancy should really be the full 39 weeks, and we now know that not only does premature delivery, elective delivery, result in those harms that require going to newborn intensive care, but we also know that those episodes of intensive care can be associated not only with disruption of what should be a joyous time in the family’s life, but with lifelong deficits in reading and mathematics. So, we knew that we had a challenge and we knew we had a responsibility to do something about that.
Chip Kahn (07:25):
Making this discovery, what’s the remediation? What did you come up with as the ways to solve or at least reduce this problem and find better outcomes?
Dr. Jon Perlin (07:37):
Well having created the evidence, we felt an obligation put that evidence into practice, and so it really required a wholesale change of clinician and frankly patient behavior. We embarked first on trying to change the physician behavior. We did a study of three different approaches to try to get physicians to use this new evidence. The first approach was that we would provide education and we showed the data. It didn’t work. The second approach that we tried was what we called soft stop, where we shared the data and the physicians agreed that afterwards they could explain why they took a mother too early elective pre-39 week delivery. It didn’t work.
The third approach, called the heart stop and now the industry standard, was one wherein the physicians agreed as part of the medical staff privileging process, that it was inappropriate to take a mother to pre 39 week delivery. And if they did, they would agree that they would sanction themselves, and that stop worked. That that approach worked, helped to change not only the practice of care in HCA, but it became a national standard in the United States, endorsed by the American College of Obstetrics and Gynecology. It was quickly adopted in other countries like Canada and quickly adopted around the world.
You know in addition to improving the outcomes for babies, there are some other ramifications that are pretty important. First for us, we felt it was important to do the right thing. It quickly reduced our newborn intensive care unit census and and changed our business. Now it wasn’t a very good business move, but it was absolutely the right thing to do. Second, we now have space for babies that really needed to be in the newborn intensive care unit and we could focus on on that. Third, as we publish this and it was adopted by other health systems, it became ultimately adopted by the Centers for Medicare and Medicaid Services. Under the Center for Medicare and Medicaid innovation, the secretary of Health and Human Services use this as a keystone of the strong start for healthy mothers and newborns program.
Why was it important to them? Because Medicaid is the payer for 40% of deliveries in the United States, and using the most conservative estimates, beyond the savings in terms of safety and potential harm to newborns, this would save US taxpayer through the Medicaid program far more than a billion dollars every year.
It takes me back relate to something I learned from Dr. Frist, our founder, Dr. Tommy Frist Junior. I remember when he was talking to a young physician in our clinical services group and he asked, “Why is what you’re doing important?” This young physician turned back to them and said, “Well, it’ll change care in HCA.” Dr. Frist is a pretty quiet guy, but he looked very expressively back of this young physician and implored him to really finish that thought. This young physician broken to smile and said, “I get it. If we change care in HCA, we change care across the United States.” Dr. Frist looked back at him again. He smiled even further. He said, “I get it. If we change care here, we change care.”
And so, this is really the privilege of scale. It’s living to our mission, the care and improvement of human life. It helps us use this incredible platform, not only to deliver great patient care here, but to advance knowledge that helps everybody.
Chip Kahn (11:07):
Jon, one of the other areas we talked about looking into was MRSA, which was an infection that usually, frequently occurs in the hospital. So, patients will come in with some other condition and unfortunately their hospital stay alone is the reason they get this infection, which is a very difficult infection to cure and frequently has all kinds of side effects that can lead to bad outcomes. You have made incredible progress here. Can you describe how the discoveries were made that led to the conclusions that MRSA could be reduced, that it was an accident in the sense that happened in the hospital that was unnecessary?
Dr. Jon Perlin (11:57):
Well, thanks for that question, Chip. You’re absolutely right. Today, we understand that so many of things that we used to think were unavoidable are in fact unavoidable. A large percentage of hospital acquired infections, including highly drug resistant staph infections, including the one we call Methicillin- resistant Staphylococcus aureus or MRSA, are known to be substantially avoidable.
Let me set the stage a little bit, because we thank you and the federation’s work for their work and really making this a national effort to avoid this avoidable harm. Across the United States, we have an epidemic. About one out of every 20 patients who goes into an American hospital will leave, if they’re fortunate enough to leave, with something that didn’t go in with, a hospital acquired infection. This results in infections in over 2 million people every year, and about 80,000 patients pay the ultimate price. They die of a hospital acquired infection. Just to put that number, 80,000 deaths from a hospital acquired infection, in perspective, that toll is actually greater than the aggregate total annually of car accidents, plus breast cancer, plus HIV combined, so it’s substantial.
We thought to ourselves what could we do to really get at this epidemic? We went to the literature and the truth is the literature didn’t have a lot of answers, so we went to the empirical evidence that is what was working around the world. As we looked out, we wondered why in the Netherlands and in Scandinavian countries, the rates of infection from methicillin resistant staph, or what we’re calling MRSA, we’re so vanishingly small. We discovered that what they did was when patients had previous healthcare encounters that could expose them to infections like MRSA, they would actually screen those patients by taking a nasal swab and a culture, and if those patients were positive for MRSA, they would be placed in isolation.
We tried that in HCA. It worked amazingly well. In fact, it got our MRSA rates down and our overall bloodstream infection rates and central line infections rates down to about a third lower than was expected based on the risk of our patients. I had the opportunity to present this at what was then called the Institute of Medicine. We we’re pretty proud of it. Afterwards, a couple of folks pulled me aside.
One, a guy named Jon Jernigan from the Centers for Disease Control and a colleague from Harvard, Rich Platt said, “Hey, you know, that’s good. But how do you know it’s really best?” Said, “What do you mean?” “When there are two other strategies, that are in equipoise, that the evidence or the empiric data suggests that they may be as good, but we don’t really know. Could a large health system that’s willing to test three different approaches actually run a fly off between these three approaches and find out which one is really best?”
The base case was what we’re doing. On admission to intensive care, we would screen the patient for MRSA with a nasal swab, and if we’re positive, we put them in isolation. Door two was do the same thing, but if they were positive, not just put them in isolation but treat their MRSA. We would give them a sponge bath with an antiseptic and a nasal antibiotic. Then door three was something that was entirely radical. It was an approach where we’d say, “Skip the culture, skip the isolation, let’s get straight to what we call decolonization, the sponge bath with an antiseptic and the nasal antibiotic.” We randomized 43 hospitals by hospital, so there’d be no patient to patient cross contamination, and over the course of 18 months enrolled 75,000 patients in a three-way comparative effectiveness study. The answer that was published in the New England Journal was that on top of all known best practices, you could cut rates of MRSA by 37%, and rates of all potentially life threatening bloodstream infections by nearly half, 44%, and this was absolutely transformative. It’s now, again, a national standard and an international standard, this approach.
But I’d say there are a couple other things. First, we got away from isolation of patients. Isolation is isolating. It’s really hard on patients, particularly in critical care. Second, you got rid of that time lag. Third, and I think the most important other headline and really gets back to the question of using data at scale, and improving at speed and at scale, is that it didn’t take a single hospital 64 years to get this data. Rather if you do the math, it took 43 hospitals 18 months. So in 18 months, we had an answer. What excites me about the future is that I hope to be able to look into, as we’re beginning to do now, our data that emerge in the context of a learning health system and be able to answer questions from the variation that may already exist so that it doesn’t take 18 months, but 18 minutes to answer important questions like these.
Chip Kahn (17:04):
This data issue you bring up Dr. Perlin, I think the work that you’re doing on Sepsis really emerges here. As we’ve been discussing, Sepsis is the kind of infection that, in a sense creeps, up on very sick patients, usually with other conditions, and historically very high death rates, and usually caught too late.
What here did you look into and what’s made a difference?
Dr. Jon Perlin (17:34):
Well, Chip, you’ve framed that absolutely correctly. Sepsis is technically defined as an overwhelming infection, but it happens in an individual who, for whatever reason, is vulnerable. Whether they’ve got other chronic illnesses or whether they’re just have our particular immune that allows this infection takeover, and Essentially it turns the body’s own immune system against itself and if not detected quickly, the patient can die very quickly. Mortality rates are on the rate of about one and three. For every hour of delayed diagnosis, looking retrospectively, the increase in mortality are between 4 and 7%.
The rate of mortality overall in the country is significant. Sepsis is the number 11 cause of death in the United States. It’s number nine cause of death in the hospital, it’s the number three cause of death in all intensive care units, and it’s the number one cause of death in noncardiac intensive care units. Well before I talk about exactly what we did, a little thought experiment.
If your loved one is in the hospital, what would you want from a clinician? Well, you’d want their full attention, 24 hours a day, seven days a week, 365 days a year. You want them aware of every new piece of lab data at the moment it was created. You’d want them to have the mathematical ability to look at the relationships of all of these data as they’re being created and see if it implies something. You’d want them to be aware of the significance of any piece of data and you want them connected to other members of the care team who could act on these data.
Well, you know, it doesn’t work that way. Your clinician can’t be with you and at the computer checking the lab data at the moment it’s created. But the computer can be programmed to follow that 247, 365. what we did is we looked at the lab data and the other markers of Sepsis and said, “Let’s train the computer to detect signal of patients as they deteriorate.” Called this program, which uses advanced analytics and data science, SPOT, Sepsis Prevention and Optimization of Therapy. But Spot of course is the name of every kid’s dog, and it’s really our Sepsis sniffer, and now it surveils basically every patient in HCA hospitals 24 hours a day, seven days a week. It’s a new system. How good is it? Well, it’s so good that it detects patients as accurately as our best clinicians when they do have Sepsis and it is actually twice as specific that it’s even more accurate by a factor of two in not detecting patients who really don’t have Sepsis.
Why is this important? Well, it’s important because every hour of delay represents an increased chance of mortality and the current state of the art before this was called the National Stop Severe Sepsis Program. It’d be like the, we’re here in Nashville, the Nashville Stop Severe Fire Program. We’re looking out this window at the buildings on the horizon, we wouldn’t intervene until we saw flames shooting out. You’d say, “That’s crazy. Why don’t we sniff smoke?” Well, we have the tools for sniffing smoke, we now have the toll for sniffing the Sepsis. And over the last three years, both that program of detecting patients at the cusp of deterioration and this new program, the SPOT sniffer for Sepsis, has saved over 5,500 of lives from rates of survival that were already better than industry, but 5,500 people.
Think about it this way. When I was down in Florida, I saw a cruise ship with a bow around it. I was wondering why the bow was on this cruise ship. It was brand new and they were celebrating the maiden voyage. And so, I saw this cruise ship on the news that evening, and talking about the fact that it helped 5,500 passengers, and I just thought about all of those patients who would live to see in a new year, to welcome the birth of a grandchild, celebrate a holiday, who otherwise wouldn’t have by virtue of the Sepsis sniffer that really helps us gain 18 hours earlier detection compared to humans in a condition for which time is really life.
Chip Kahn (21:47):
Is this being disseminated?
Dr. Jon Perlin (21:49):
Yeah. It is absolutely being disseminated. We are live across almost all HTA hospitals. By the end of the third quarter of this year, every last facility that’s been part of the HCA family for more than a year will have this up and running, but we have now had this monitoring over a million inpatients. The nurses and the doctors just tell us that. “Yeah, there are some patients that had detected that we would have known about,” but importantly they’ve told us time and again, there are patients we didn’t appreciate we’re headed towards Sepsis.
Chip Kahn (22:21):
Dr. Perlin, so we’ve outlined here three examples, extremely meaningful examples of using data and analysis to change care and obviously lead to better outcomes for patients. Where do you think we’ll go next with big data, what’s the future, and can we speed up these processes so we can learn more to care better?
Dr. Jon Perlin (22:50):
This is a great question, Chip. I think now that we’ve had some first use cases on how we can harness data to learn it at speed and at scale, I think we can begin to look into the data to do things in the computer that we would’ve had to have set up a trial to do in the past.
A lot of people say they want to eliminate variation. I don’t want to eliminate variation and regress to the mean, I want to use variation to discover practices at the high end. Why do some patients who are treated in a particular way do better? How can we harvest that signal and then reduce the variation around that new best practice? And that’s where we’ll go in terms of using this inevitable data byproduct of care for the help of discovery. It’s really the ability to systematically look into the collective memory of everything that we’re doing in healthcare to improve care.
So what are the next areas? Well, we hear a lot about artificial intelligence where machines emulate human thought processes to help make better decisions. We hear a lot about natural language processing where computers read charts, I naturally say charts in healthcare because the chart or the electronic record is, in fact, about 20% structured data and about 70 to 80% unstructured or text data. There’s this incredible richness of information that we’re systematically neglecting because it’s easier to get at the computable stuff, the numbers, than it is to get at the meaning of the text.
We’re harnessing that. Let me give you a couple of examples of how we’re using that. Chip, you’ve done a lot of work with the National Academy of Medicine, and you’ve worked with them since your time in Congress, and since the publication of the seminal safety report To Err Is Human. Well not as sexy a title, but the most recent report in the series that began with To Err Is Human was one called Improving Medical Diagnosis. It was really about the harms of misdiagnosis and missed, M-I-S-S-E-D diagnosis. I bet every one of us has a story of a friend, a relative, an acquaintance who had something missed and had a bad outcome.
In fact, one of my favorite cousins had an X ray that in fact ruled out of fracture from a bike accident, but no one followed up on what otherwise would have been early, treatable lung cancer. He died about six months later when the pain was overwhelming, and that wasn’t the incidental finding, that was the finding. In this report for the National Academy of Medicine, they note that missed incidental findings or one of the leading causes of death. They’re certainly one of the leading causes of malpractice suits against emergency departments.
What if you could actually train a computer, using machine learning, that is the iterative feeding of data so that it recognizes a pattern, and using natural language processing, the ability to interpret the written text, to separate out those imaging studies in which there was an important incidental finding, like an early operable, curable lung cancer?
Well, this is what we did. Using three HCA hospitals a couple of years ago, we train the system to understand the difference for example, between tobacco farmer and tobacco user, tobacco farmer having no higher risk, tobacco user obviously having a higher rest. And so over the course of the 13 months, the three emergency departments generated about 33,000 CTs. We have those CTs reviewed by radiologists, we also had the machine that was trained to read the imaging reports make a decision about which ones had an incidental finding. They agreed on approximately the same 1055 images had something suspicious. We then had a protocol that both followed to identify which ones likely needed a follow-up or a biopsy, and they agreed on the next 1155 or so that needed some follow up. Then out of that, I can tell you that there were 58 positive findings from the biopsies that follow that follow-up, and a third of those were early curable lung cancers.
So, we’re now rolling out a program to detect incidental findings of the sort so that we can, on the downside, never miss another incidental finding and hopefully set a new standard for safety, and on the upside, identify early operable, curable disease. We think that this type of use of artificial intelligence, machine learning, and natural language processing helps usher in a new era of quality and patient safety.
Chip Kahn (27:44):
Dr. Perlin, thank you so much for this interesting discussion, and I think particularly what I take away is in a sense not what you have achieved, but the exciting possibilities for the future of what will come next. Thanks so much.
Dr. Jon Perlin (28:01] Thank you, Chip. Privilege to be with you today):
Chip Kahn (28:03):
Thanks for listening to Hospitals in Focus. Please be sure to subscribe to us on Apple podcasts, Google podcasts, your favorite podcast platform, or by visiting us at our website, FAH.org. We hope you will share your reviews and tell a friend about us. Thanks so much for listening.
Dr. Jon Perlin